Preventable heart disease is one of the major causes of death in the world. The World Health Organization estimates that cardiovascular disease caused 31% of all deaths in 2016, making it the number 1 cause of death globally¹. Among cardiovascular diseases, cardiac arrhythmia is one of the most common. Arrhythmia describes a set of conditions in which the heart beats too quickly, too slowly, or with an irregular pattern. Arrhythmias are responsible for up to 80% of sudden cardiac arrest cases which leads to around 12% of all deaths in a year.
Whereas some forms of cardiovascular disease are fully preventable through lifestyle changes like increased physical activity and better nutrition, other conditions, like atrial fibrillation (AFib), are more challenging to recognize. This is the most common form of heartbeat irregularity, and where there is hope that new monitoring tools can significantly improve our ability to understand and prevent the disease.
AFib happens when the two upper chambers of the heart (atria) don’t work in sync with the lower chambers, contracting randomly. When this happens, the lower chambers do not fill completely or pump enough blood to the lungs and body. AFib puts patients at an increased risk for stroke, death, heart failure, and dementia. But this arrhythmia often is hard to detect due to a lack of noticeable symptoms. If the AFib episodes are intermittent or infrequent, a patient might have to wear a monitoring device continuously for days or even weeks, until an event is detected.
That is why the possibility of continuously recording heart rate and rhythm via an everyday wearable, like a smartwatch, is appealing to cardiologists. It raises the possibility of non-invasive extended arrhythmia monitoring. Once linked with the smartwatch, software could detect an irregular rhythm and alert the user or even send the data to a cardiology reporting service for further analysis. Event triggers could be linked with other sensors data like GPS and gyroscopes/accelerometers to correlate symptom timing with the wearers activity.
Heart rate sensors on most smartwatches work via photoplethysmography (PPG): the technology used by conventional pulse oximeters to measure the heartbeat via monitoring color changes — and hence light absorption — at the skin surface.
There are many studies of AFib detection from electrocardiogram (ECG) signals. However, since PPG is a noninvasive technique and can be recorded on most modern devices, it shows great promise as an alternative to ECG-based solutions. Studies show that continuous monitoring with smart-device based PPG technology could be a feasible, cost-effective approach for AFib screening³. This would help efforts at screening and detection of AFib, as well as early interventions to reduce stroke and other AFib-related complications.
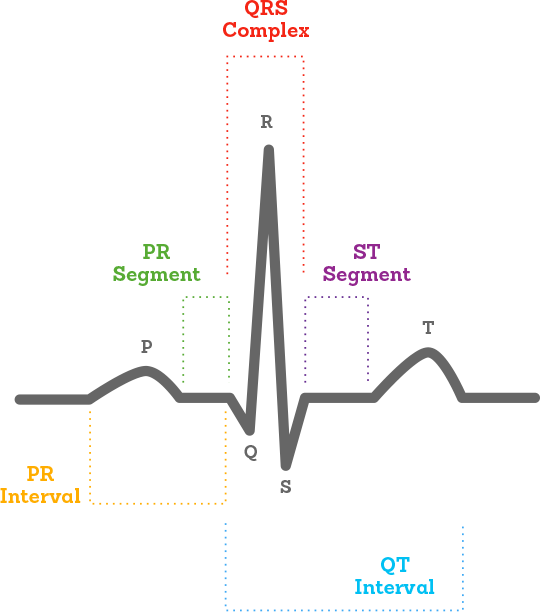
ECG (electrocardiopgrah) is a visual representation of the electric signals originating from the heart.
PPG (photoplethysmogram) uses light sensors to measure the changes in volume of the capillaries under the skin. An LED shines light against the skin; some of it is reflected and scattered back onto the photodiode; the amount that is reflected changes as the heart beats, which determines the heart rate.
But current devices are not without limitations. They need to be recharged on a daily basis, thus limiting the potential monitoring time to daylight hours only. Furthermore, changes in skin color, tattoos, temperature, erratic movement, and variations in skin pressure or even hairy wrists as well as fluctuations in oxygen levels can all affect readings via PPG⁴.
There is also the matter of privacy. The increased transfer of health information from consumers to private companies raises important questions about the ownership of such data. If such physiological information is transmitted and collected continuously, with whom would the ownership of the information reside?
Smart software and algorithms play a significant role in aiding decision-making among health practitioners along the continuum of prevention, diagnosis, treatment and patient follow-up. Machine Learning permits early, accurate diagnosis by aggregating disparate pieces of information, extracting key patterns from datasets to help identify effective interventions early on when conditions are amenable to treatment. The challenge is to do it in a way that is accurate, fair, transparent and that doesn’t compromise the patient’s privacy.
Over the following posts in this series, I’ll explore how to use Convolutional Neural Networks to detect AFib from ECG data; how to do it in a privacy-preserving way with Differential Privacy and Homomorphic Encryption; and how to use Computer Vision and Augmented Reality to make some of the information visible in interesting ways.
At 1D.works we’re excited about the potential of AI to improve businesses and people’s lives. Healthcare is just one of the largely unexplored territories we’re invested in. If you think you can benefit from a decade-long experience of applying machine learning to business processes.
[1] https://www.who.int/en/news-room/fact-sheets/detail/cardiovascular-diseases-(cvds)
[2] R. Mehra, “Global public health problem of sudden cardiac death,” Journal of electrocardiology, vol. 40, pp. S118–S122, 2007.
[3] http://www.onlinejacc.org/content/74/19/2365
[4] Scully CG, Lee J, Meyer J, Gorbach AM, Granquist-Fraser D, Mendelson Y et al. . Physiological parameter monitoring from optical recordings with a mobile phone. IEEE Trans Biomed Eng 2012;59:303–6.